|
|
Abstract: 
Short Biography: Prof. Juan A. Cuesta-Albertos (Segovia, Spain, 1955) has a degree in Mathematics, specializing in Statistics and Operations Research, from the University of Valladolid, Spain. He completed his doctoral thesis at the same university, in Mathematical Sciences, under the direction of Prof. Dr. D. Miguel Martín Díaz. He taught at the Colegio Universitario de Burgos until the 1981/82 academic year, and from that moment until the present day, at the University of Cantabria, where he is a Full Professor of Statistics. He is the author of about 85 research papers on topics such as the laws of large numbers and centralization measures in abstract spaces, bootstrap, robustness, functional data analysis, etc. Apart from continuing his research work, he has recently been paying attention to the dissemination of statistics. He was the Chief Editor of the journal TEST from 2002 to 2004, and is currently an Associate Editor of JASA. Please find more details in this link: https://personales.unican.es/cuestaj/index.html.
Abstract: 
Short Biography: Dr. Mallesham K completed his MSc in Mathematics from IIT Madras in 2011, and joined Harish-Chandra Research Institute (HRI), Allahabad, as PhD student. He received his PhD degree from HRI in September, 2018. Currently, he is a visiting scientist in the Statistics and Mathematics Unit, Indian Statistical Institute, Kolkata. His research area of interest lies in Number theory, especially in Analytic number theory.
Abstract: 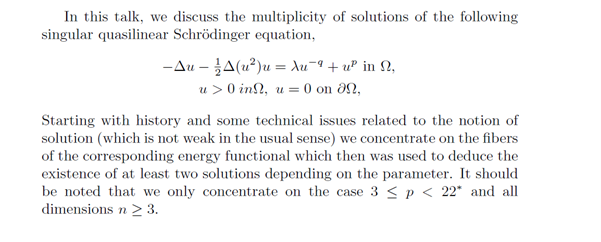
Abstract: 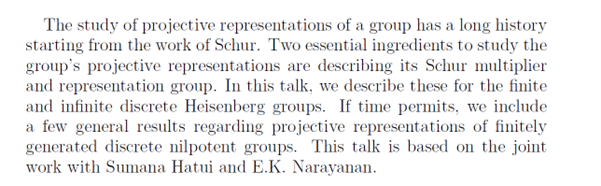
Abstract: 
Abstract: 
Abstract: 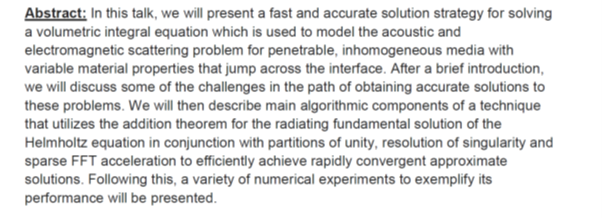
Abstract: 
Abstract: 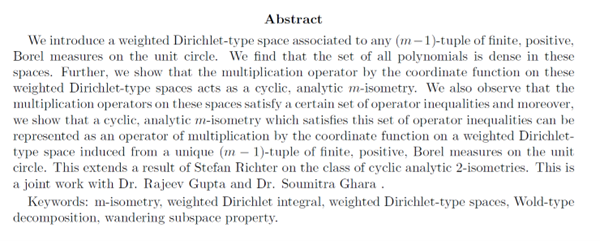
Short Biography: Dr. Ramiz Reza completed his B.Sc. from Ramakrishna Mission Vidyamandira, Belur (University of Calcutta) in 2009. After that, he obtained his Integrated Ph.D. degree (both M.S. and Ph.D.) from Indian Institute of Science, Bangalore in 2017. He started his post-doctoral research as a SERB-NPDF fellow in IISER Kolkata in September 2017. In February 2018, he moved to Chalmers University of Technology, Gothenburg, Sweden to continue his post-doctoral studies as a SERB-OPDF fellow. Currently, he is an institute postdoctoral fellow at Indian Institute of Technology, Kanpur (since May 2019).
Abstract: 
Short Biography: Dr. Kumar completed his graduation in Mathematics from C.C.S. University Meerut in 2012, his post-graduation in Mathematics from IIT Guwahati in 2014, and Ph.D. in Mathematics from NISER, Bhubaneswar under the supervision of Dr. Anil Kumar Karn in 2020. He is currently working as an adhoc faculty in School of Sciences (Mathematics), NIT, Andhra Pradesh.
Abstract: 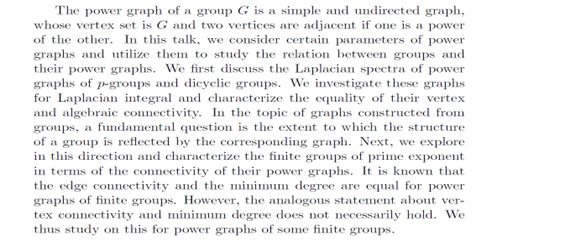
Short Biography: Dr. Panda is currently a Post Doctoral Fellow at the School of Mathematical Sciences, NISER, Bhubaneswar. He obtained his B.Sc. from Gangadhar Meher Autonomous College, Sambalpur; M.Sc. from IIT Kanpur and Ph.D. from IIT Guwahati (all in Mathematics). His main research interest is algebraic combinatorics.
Abstract: 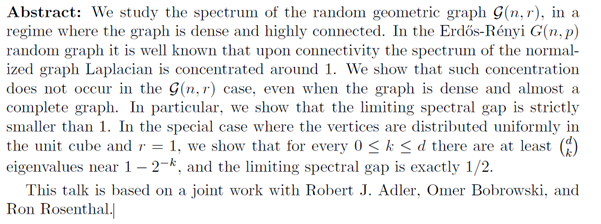
Short Biography: Dr. Adhikari is currently a Viterbi and Zeff Post Doctoral Fellow at the Department of Electrical Engineering, Technion, Israel. Prior to that, he was a Post Doctoral Fellow at Indian Statistical Institute, Kolkata. He obtained both degrees M.S. and Ph.D. (in Mathematics) from Indian Institute of Science, Bangalore. He completed his Bachelor degree (Mathematics honours) from Ramakrishna Mission Residential College, Narendrapur (under Calcutta University). His research interests mainly lie in probability theory and analysis, specifically, random matrix, determinantal point processes, large deviation, Steins method for normal approximation, and potential theory, free probability, stochastic geometry and random graphs.
Abstract: such as medical imaging and geophysical imaging. First half of the talk will be a brief overview of our work related to introduced transforms and the second half will be focused on a recent work on V-line tomography for vector fields in
Abstract: 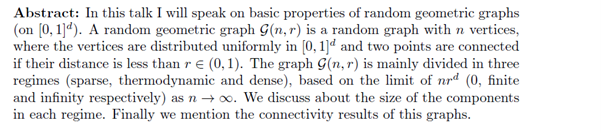
Short Biography: Dr. Adhikari is currently a Viterbi and Zeff Post Doctoral Fellow at the Department of Electrical Engineering, Technion, Israel. Prior to that, he was a Post Doctoral Fellow at Indian Statistical Institute, Kolkata. He obtained both degrees M.S. and Ph.D. (in Mathematics) from Indian Institute of Science, Bangalore. He completed his Bachelor degree (Mathematics honours) from Ramakrishna Mission Residential College, Narendrapur (under Calcutta University). His research interests mainly lie in probability theory and analysis, specifically, random matrix, determinantal point processes, large deviation, Steins method for normal approximation, and potential theory, free probability, stochastic geometry and random graphs.
Abstract: The aim of this talk is to prove a very important result of analysis named the Fundamental Theorem of Calculus (FTC) which basically relates the concepts of integration and differentiation. To achieve this
Short Biography: Dr. Mishra received his PhD in 2017 under the guidance of Dr. Venky P. Krishnan from TIFR Centre for Applicable Mathematics, Bangalore, India. His primary research interests are in the field of inverse problems related to integral geometry, partial differential equations, microlocal analysis and medical imaging. Currently, he is a postdoctoral scholar in the Department of Mathematics at the University of Texas at Arlington (UTA), Texas. Before joining UTA, he was a postdoctoral scholar at the University of California, Santa Cruz from August 2017 to July 2019.
Abstract: We have established limit theorems for the sums of dependent Bernoulli random variables. Here each successive random variable depends on previous few random variables. A previous k-sum dependent model is considered. This model is a combination of the previous all sum and the previous k-sum dependent models. The law of large numbers, the central limit theorem and the law of iterated logarithm for the sums of random variables following this model are established. A new approach using martingale differences is developed to prove these results.
Short Biography: Currently, Dr. Singh is working at Redpine Signals India as a Research Engineer, where he is exploring the mathematical concept of Quantum Machine Learning Algorithms. He has completed his Ph.D. in Probability Theory under the supervision of Prof. Somesh Kumar in June, 2020. During his Ph.D., he also served as a Teaching Assistant for NPTEL courses for three years.
Abstract: We have established limit theorems for the sums of dependent Bernoulli random variables. Here each successive random variable depends on previous few random variables. A previous k-sum dependent model is considered. This model is a combination of the previous all sum and the previous k-sum dependent models. The law of large numbers, the central limit theorem and the law of iterated logarithm for the sums of random variables following this model are established. A new approach using martingale differences is developed to prove these results.
Short Biography: Currently, Dr. Singh is working at Redpine Signals India as a Research Engineer, where he is exploring the mathematical concept of Quantum Machine Learning Algorithms. He has completed his Ph.D. in Probability Theory under the supervision of Prof. Somesh Kumar in June, 2020. During his Ph.D., he also served as a Teaching Assistant for NPTEL courses for three years.
Abstract: The aim of this talk is to prove a very important result of analysis named the Fundamental Theorem of Calculus (FTC) which basically relates the concepts of integration and differentiation. To achieve this
Short Biography: Dr. Mishra received his PhD in 2017 under the guidance of Dr. Venky P. Krishnan from TIFR Centre for Applicable Mathematics, Bangalore, India. His primary research interests are in the field of inverse problems related to integral geometry, partial differential equations, microlocal analysis and medical imaging. Currently, he is a postdoctoral scholar in the Department of Mathematics at the University of Texas at Arlington (UTA), Texas. Before joining UTA, he was a postdoctoral scholar at the University of California, Santa Cruz from August 2017 to July 2019.
Abstract: 
Short Biography: Dr. Adhikari is currently a Viterbi and Zeff Post Doctoral Fellow at the Department of Electrical Engineering, Technion, Israel. Prior to that, he was a Post Doctoral Fellow at Indian Statistical Institute, Kolkata. He obtained both degrees M.S. and Ph.D. (in Mathematics) from Indian Institute of Science, Bangalore. He completed his Bachelor degree (Mathematics honours) from Ramakrishna Mission Residential College, Narendrapur (under Calcutta University). His research interests mainly lie in probability theory and analysis, specifically, random matrix, determinantal point processes, large deviation, Steins method for normal approximation, and potential theory, free probability, stochastic geometry and random graphs.
Abstract: such as medical imaging and geophysical imaging. First half of the talk will be a brief overview of our work related to introduced transforms and the second half will be focused on a recent work on V-line tomography for vector fields in
Abstract: 
Short Biography: Dr. Adhikari is currently a Viterbi and Zeff Post Doctoral Fellow at the Department of Electrical Engineering, Technion, Israel. Prior to that, he was a Post Doctoral Fellow at Indian Statistical Institute, Kolkata. He obtained both degrees M.S. and Ph.D. (in Mathematics) from Indian Institute of Science, Bangalore. He completed his Bachelor degree (Mathematics honours) from Ramakrishna Mission Residential College, Narendrapur (under Calcutta University). His research interests mainly lie in probability theory and analysis, specifically, random matrix, determinantal point processes, large deviation, Steins method for normal approximation, and potential theory, free probability, stochastic geometry and random graphs.
Abstract: 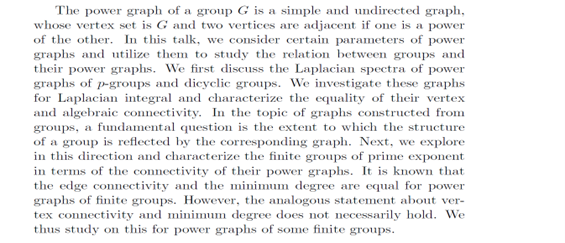
Short Biography: Dr. Panda is currently a Post Doctoral Fellow at the School of Mathematical Sciences, NISER, Bhubaneswar. He obtained his B.Sc. from Gangadhar Meher Autonomous College, Sambalpur; M.Sc. from IIT Kanpur and Ph.D. from IIT Guwahati (all in Mathematics). His main research interest is algebraic combinatorics.
Abstract: 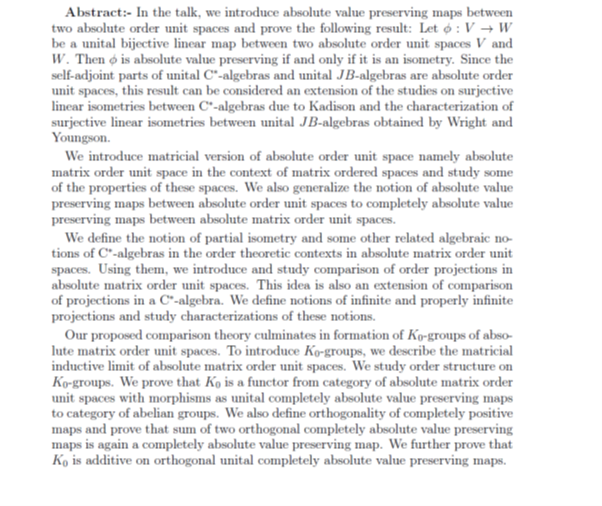
Short Biography: Dr. Kumar completed his graduation in Mathematics from C.C.S. University Meerut in 2012, his post-graduation in Mathematics from IIT Guwahati in 2014, and Ph.D. in Mathematics from NISER, Bhubaneswar under the supervision of Dr. Anil Kumar Karn in 2020. He is currently working as an adhoc faculty in School of Sciences (Mathematics), NIT, Andhra Pradesh.
Abstract: 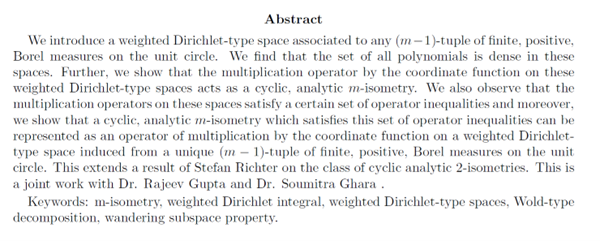
Short Biography: Dr. Ramiz Reza completed his B.Sc. from Ramakrishna Mission Vidyamandira, Belur (University of Calcutta) in 2009. After that, he obtained his Integrated Ph.D. degree (both M.S. and Ph.D.) from Indian Institute of Science, Bangalore in 2017. He started his post-doctoral research as a SERB-NPDF fellow in IISER Kolkata in September 2017. In February 2018, he moved to Chalmers University of Technology, Gothenburg, Sweden to continue his post-doctoral studies as a SERB-OPDF fellow. Currently, he is an institute postdoctoral fellow at Indian Institute of Technology, Kanpur (since May 2019).
Abstract: 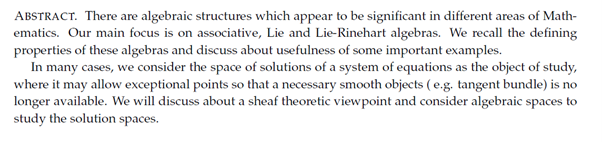
Abstract: 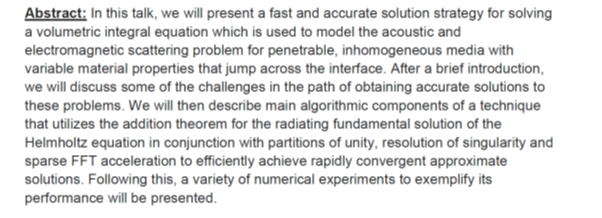
Abstract: 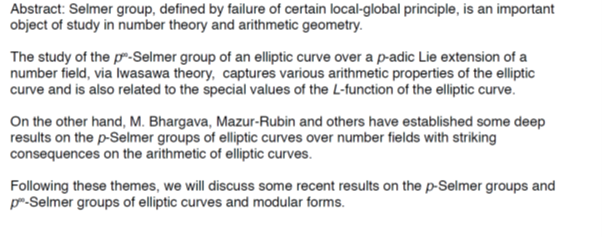
Abstract: 
Abstract: 
Abstract: 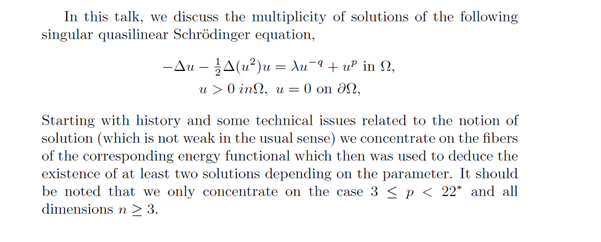
Abstract: 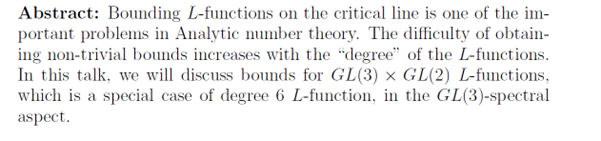
Short Biography: Dr. Mallesham K completed his MSc in Mathematics from IIT Madras in 2011, and joined Harish-Chandra Research Institute (HRI), Allahabad, as PhD student. He received his PhD degree from HRI in September, 2018. Currently, he is a visiting scientist in the Statistics and Mathematics Unit, Indian Statistical Institute, Kolkata. His research area of interest lies in Number theory, especially in Analytic number theory.
Abstract: 
Short Biography: Prof. Juan A. Cuesta-Albertos (Segovia, Spain, 1955) has a degree in Mathematics, specializing in Statistics and Operations Research, from the University of Valladolid, Spain. He completed his doctoral thesis at the same university, in Mathematical Sciences, under the direction of Prof. Dr. D. Miguel Martín Díaz. He taught at the Colegio Universitario de Burgos until the 1981/82 academic year, and from that moment until the present day, at the University of Cantabria, where he is a Full Professor of Statistics. He is the author of about 85 research papers on topics such as the laws of large numbers and centralization measures in abstract spaces, bootstrap, robustness, functional data analysis, etc. Apart from continuing his research work, he has recently been paying attention to the dissemination of statistics. He was the Chief Editor of the journal TEST from 2002 to 2004, and is currently an Associate Editor of JASA. Please find more details in this link: https://personales.unican.es/cuestaj/index.html.
Abstract: 
Short Biography: Subhabrata (Subho) Majumdar is a Senior Inventive Scientist in the Data Science and AI Research group of AT&T Labs. His research interests have two focus areas - (1) statistical machine learning - specifically predictive modelling and complex high-dimensional inference, and (2) trustworthy machine learning methods, with emphasis on human-centric qualities such as social good, robustness, fairness, privacy protection, and causality. Subho has a PhD in Statistics from the School of Statistics, University of Minnesota under the guidance of Prof. Anshu Chatterjee. His thesis was on developing inferential methods based on statistical depth functions, focusing on robust dimension reduction and variable selection. Before joining AT&T, Subho was a postdoctoral researcher at the University of Florida Informatics Institute under Prof. George Michailidis. Subho has extensive experience in applied statistical research, with past and present collaborations spanning diverse areas like statistical chemistry, public health, behavioral genetics, and climate science. He recently co-founded the Trustworthy ML Initiative (TrustML), to bring together the community of researchers and practitioners working in that field, and lower barriers to entry for newcomers. Link to his webpage: https://shubhobm.github.io/.
Abstract: Applications of Singular perturbation and its related boundary layer phenomena are very common in today's literature. Presence of a small parameter in the differential equation changes the behavior of the solution rapidly. Uniform meshes are inadequate for the convergence of numerical solution. The aim of the present talk is to consider the adaptive mesh generation for singularly perturbed differential equations based on moving mesh strategy. I shall start this talk with a small introduction on singular perturbation. The analytical and computational difficulties on the existed methods will be discussed. The concept of moving mesh strategy will be explained. For a posteriori based convergence analysis, a system of nonlinear singularly perturbed problems will be considered. In addition, the difference between a priori and a posteriori generated meshes and the effectivity of a posteriori mesh on the present research will be discussed. The parameter independent a priori based convergence analysis for a parabolic convection diffusion problem will be presented. In addition, an approach.
Abstract: In this talk, definitions of different types of cross-sectional dependence and the relations between them would be discussed. We then look into parameter estimation and their asymptotic properties.
Abstract: I will discuss on the two sections of Numerical Analysis- numerical root finding methods and interpolation techniques. I will start with the introduction on these two topics and the restriction of the popular methods. I will also discuss about interpolation and their applications.
Abstract: 
Abstract: 
Abstract: In this series of two talks, we will first define long memory processes and discuss practical and theoretical examples of such processes. In addition we shall discuss the uniform reduction principle for such processes and some of its implications. This principle says that for long memory moving average processes the suitably standardized empirical process converges weakly to a degenerate Gaussian process. This is completely unlike what happens in the independent or weakly dependent case, where the suitably standardized empirical process converges weakly to Brownian bridge. In the second talk we shall discuss the problem of fitting a known d.f. or density
to the marginal error distribution of a stationary long memory moving-average process when its mean is known and unknown. When the mean is unknown and estimated by the sample mean, the first-order difference between the residual empirical and null distribution functions is asymptotically degenerate at zero. Hence, it cannot be used to fit a distribution up to an unknown mean. We show that by using a suitable class of estimators of the mean, this first order degeneracy does not occur. We also present some large sample properties of the tests based on an integrated squared-difference between kernel-type error density estimators and the expected value of the error density estimator. The asymptotic null distributions of suitably standardized test statistics are shown to be chi-square with one degree of freedom in both cases of known and unknown mean. This is totally unlike the i.i.d. errors set-up where such statistics are known to be asymptotically normally distributed.
An interested person may find the following two references helpful.
Giraitis, L., Koul, H.L. and Surgailis, D. (2012). Large sample inference for long memory processes. Imperial College Press and World Scientific.
Koul, H.L., Mimoto, N. and Surgailis, D. (2013). Goodness-of-fit tests for long memory moving average marginal density. Metrika, 76, 205-224.
Abstract: 
Abstract: In this series of two talks, we will first define long memory processes and discuss practical and theoretical examples of such processes. In addition we shall discuss the uniform reduction principle for such processes and some of its implications. This principle says that for long memory moving average processes the suitably standardized empirical process converges weakly to a degenerate Gaussian process. This is completely unlike what happens in the independent or weakly dependent case, where the suitably standardized empirical process converges weakly to Brownian bridge. In the second talk we shall discuss the problem of fitting a known d.f. or density
to the marginal error distribution of a stationary long memory moving-average process when its mean is known and unknown. When the mean is unknown and estimated by the sample mean, the first-order difference between the residual empirical and null distribution functions is asymptotically degenerate at zero. Hence, it cannot be used to fit a distribution up to an unknown mean. We show that by using a suitable class of estimators of the mean, this first order degeneracy does not occur. We also present some large sample properties of the tests based on an integrated squared-difference between kernel-type error density estimators and the expected value of the error density estimator. The asymptotic null distributions of suitably standardized test statistics are shown to be chi-square with one degree of freedom in both cases of known and unknown mean. This is totally unlike the i.i.d. errors set-up where such statistics are known to be asymptotically normally distributed.
An interested person may find the following two references helpful.
Giraitis, L., Koul, H.L. and Surgailis, D. (2012). Large sample inference for long memory processes. Imperial College Press and World Scientific.
Koul, H.L., Mimoto, N. and Surgailis, D. (2013). Goodness-of-fit tests for long memory moving average marginal density. Metrika, 76, 205-224.
Abstract: Lie algebras are infinitesimal counterpart of Lie groups. In fact, there is a one-to-one correspondence between simply-connected Lie groups and Lie algebras (Lie III theorem).
In this talk, we will introduce 'multiplicative Poisson structures' on Lie groups (called Poisson-Lie groups) and 'Lie bialgebra' structures on Lie algebras. Finally, we show that there is a one-to-one correspondence between Poisson-Lie groups and Lie bialgebras. If time permits, I will mention some generalizations of the above correspondence.
Abstract: While studying the dynamics of polynomial automorphisms in C2, it turns out that the class of Hénon maps, which exhibits extremely rich dynamical behaviour, is the single most important class to study. An extensive research has been done in this direction by many authors over the past thirty years. In this talk, we shall see a `rigidity' property of Hénon maps which essentially replicates a classical `rigidity' theorem of Julia sets of polynomial maps in the complex plane. In particular, we shall give an explicit description of the automorphisms in C2 which preserve the Julia sets of a given Hénon map. If time permits, we shall see a few more results regarding `rigidity' property of some special classes of automorphisms in higher dimension.
Abstract: Natural language processing (NLP) has become a hot topic of research since the big five (GAFAM) are investing heavily into the development of this field. NLP tasks are wide-ranging, e.g. machine translation, auto completion, spam detection, argument mining, text classification, sentiment analysis, chat bots and named entity recognition. In any case, the text has to be converted somehow into numbers to use the text e.g. in classification problems.
The talk will be split into two parts. In the first part, the problem is introduced and the necessary preprocessing steps as well as simple methods for the representation of documents are described. These methods allow, e.g., to include text documents as features in modern machine learning methods used for classification and regression (supervised learning). The second part introduces the so-called neural language models which are unsupervised methods to “learn” a language. This results in embedding words and documents using so-called word or document vectors (e.g. word2vec). A disadvantage is that these vectors are not context sensitive. Therefore, we also give an outlook to the newest, usually very large, language models (based on deep learning methods) which are often freely available and can therefore be used by everybody for own purposes.
Abstract: Current tools for multivariate density estimation struggle when the density is concentrated near a nonlinear subspace or manifold. Most approaches require choice of a kernel,with the multivariate Gaussian by far the most commonly used. Although heavy-tailed and skewed extensions have been proposed, such kernels cannot capture curvature in the support of the data. This leads to poor performance unless the sample size is very large relative to the dimension of the data. This article proposes a novel generalization of the Gaussian distribution, which includes an additional curvature parameter. We refer to the proposed class as Fisher-Gaussian (FG) kernels, since they arise by sampling from a von Mises-Fisher density on the sphere and adding Gaussian noise. The FG density has an analytic form,and is amenable to straightforward implementation within Bayesian mixture models using Markov chain Monte Carlo. We provide theory on large support, and illustrate gains relative to competitors in simulated and real data applications.
Abstract: T11 Target structure (T11TS), a membrane glycoprotein isolated from sheep erythrocytes, reverses the immune suppressed state of brain tumor induced animals by boosting the functional status of the immune cells. This study aims at aiding in the design of more efficacious brain tumor therapies with T11 target structure. In my talk, I will discuss about the dynamics of brain-tumor immune interaction though a system of coupled non-linear ordinary differential equations. The system undergoes sensitivity analysis to identify the most sensitive parameters. In the model analysis, I obtained the criteria for the threshold level of T11TS for which the system will be tumor-free. Computer simulations were used for model verification and validation.
Abstract: Computationally determinant and permanent of matrices are the two extreme problems. The determinant is in P whereas the permanent is a #P- complete problem, which means that the permanent cannot be computed in polynomial time unless P = NP. In this talk, we will discuss how the determinant (permanent) of a matrix can be computed in terms of the determinant (permanent) of blocks in the corresponding digraph. Under some conditions on the number of cut-vertices and block sizes the computation beats the asymptotic complexities of the state of art methods. Next, as an application of the computation of determinant using blocks, we will discuss a characterization of nonsingular block graphs which was an open problem, proposed in 2013 by Bapat and Roy.
Abstract: I will discuss a discrete scattering problem which can be reduced to a 2x2 matrix factorization of Wiener-Hopf on an annulus (including the unit circle) in complex plane. An advancement made last year will be also presented that effectively solves this factorization problem but in an intricate manner. The particular factorization problem is still open from point of view of explicit factors and corresponds to an analogous problem on an infinite strip (including the real line) in complex plane (also open since several decades).
Short Biography: Dr. Arkaprava Roy is currently a postdoctoral associate at Duke University, currently working with Dr. David Dunson. I have completed my Ph.D. in statistics in April 2018 from North Carolina State University (NCSU), under the supervision of Dr. Subhashis Ghosal and Dr. Ana-Maria Staicu after a Bachelor and Masters degree in statistics from Indian Statistical Institute, Kolkata. My focus is on data science and in developing innovative statistical modeling frameworks and corresponding inference methodology motivated by complex applications. I will be joining University of Florida, Biostatistics department in June, 2020 as an Assistant Professor.
Abstract:
Short Biography: Dr. Ravitheja Vangala did his B.Math. from ISI Bangalore, M.Sc. in Mathematics from CMI Chennai, and Ph.D.in Mathematics from TIFR Mumbai. He works in number theory. His current research interests are reductions of local Galois representations, modular representation theory, p-adic L-functions and Iwasawa theory.
Abstract: In 1961, James and Stein introduced an estimator of the mean of a multivariate normal distribution that achieves a smaller mean squared error than the maximum likelihood estimator in dimensions three and higher. This was in fact a surprising result by Stein. I will discuss the proof of this result. After that I will discuss some other estimators which are competitors of the James - Stein estimator and if the time permits the James-Stein estimator as an Empirical Bayes Estimator will also be discussed.
Abstract: In this long talk, motivated by the need to deal with free boundary or interface problems of practical engineering applications, a variety of numerical methods will be presented. Apart from isogeometric methods (and its variants), we provide a primer to various numerical methods such as meshfree methods, cutFEM methods, and collocation methods based on Taylor series expansions. We review traditional methods and recent ones which appeared in The last decade for a variety of applications.
Abstract: In this talk, the first a posteriori error-driven adaptive finite element approach for real-time surgical simulation will be presented, and the method will be demonstrated on needle insertion problems. For simulating soft tissue deformation, the refinement strategy relies upon a hexahedron-based finite element method, combined with a posteriori error estimation driven local h-refinement. The local and global error levels in the mechanical fields (e.g., displacement or stresses) are controlled during the simulation. After showing the convergence of the algorithm on academic examples, its practical usability will be demonstrated on a percutaneous procedure involving needle insertion in a liver and brain. The brain shift phenomena is taken in to account which occurs when a craniotomy is performed. Through academic and practical examples it will be demonstrated that our adaptive approach facilitates real-time simulations. Moreover, this work provides a first step to discriminate between discretization error and modeling error by providing a robust quantification of discretization error during simulations. The proposed methodology has direct implications in increasing the accuracy, and controlling the computational expense of the simulation of percutaneous procedures such as biopsy, brachytherapy, regional anaesthesia, or cryotherapy. Moreover, the proposed approach can be helpful in the development of robotic surgeries because the simulation taking place in the control loop of a robot needs to be accurate, and to occur in real time. The talk will conclude with some discussion on future outlook towards personalised medicines.
Abstract: In this talk, we will discuss about the boundedness of the conformal composition operators on Besov spaces defined on domains in the Euclidean plane. We will see how the regularity of domains effect the boundedness of the operators. The related open problems will also be discussed.
Abstract: In binary classification, results from multiple diagnostic tests are often combined in many ways like logistic regression, linear discriminant analysis to improve diagnostic accuracy. In recent time, combining methods like direct maximization of the area under the ROC curve (AUC) has received significant interest among researchers in the field of medical science. In this article, we develop a combining method that maximizes a smoothing approximation of the hyper-volume under manifolds (HUM), an extended notion of AUC when disease outcomes are multi-categorical with ordinal in nature. The proposed method is distribution-free as it does not assume any distribution of the biomarkers. Consistency and asymptotic normality of the proposed method are established. The method is illustrated using simulated data sets as well as two real medical data sets.
Abstract: Recent years have witnessed tremendous activity at the intersection of statistics, optimization and machine learning. The consequent bi-directional flow of ideas has significantly improved our understanding of the computational aspects of different statistical learning tasks. Our talk presents new results for two fundamental tasks at the intersection of statistics and optimization namely, (i) efficiently generating random samples given partial knowledge of a probability distribution, and (ii) learning parameters of an unknown distribution given samples from it. In the first part of the talk, we present non-asymptotic convergence guarantees of several popular Monte Carlo Markov Chain (MCMC) algorithms including Langevin algorithms and Hamiltonian Monte Carlo (HMC). We also underline beautiful connections between optimization and sampling, which leads to a design of faster sampling algorithms. In the second part of the talk, we present nonasymptotic results for parameter estimation of mixture models given samples from the distribution. In particular, we provide algorithmic and statistical guarantees for the Expectation-Maximization (EM) algorithm when the number of components is incorrectly specified by the user.
|